Stochastic Processes: Data Analysis and Computer Simulation
Start_Stochastic Presesses Ryoichi Yamamoto
-
- Start_Stochastic Presesses
-
Ryoichi Yamamoto
Feb. 20, 2023 02:45 English
-
- week1-1_Using Python iPython and Jupyter notebook
-
Ryoichi Yamamoto
Feb. 20, 2023 08:37 English
-
- week1-2_Making graphs with matplotlib
-
Ryoichi Yamamoto
Feb. 20, 2023 07:30 English
-
- week1-3_The Euler method for numerical integration
-
Ryoichi Yamamoto
Feb. 20, 2023 10:37 English
-
- week1-4_Simulating a damped harmonic oscillator
-
Ryoichi Yamamoto
Feb. 20, 2023 07:44 English
-
- week2-1_Stochastic variables and distributionnfunctions
-
Ryoichi Yamamoto
Feb. 20, 2023 20:40 English
-
- week2-2_Gennerationg random numbers with Gaussian_binomiak_Poisson distributions
-
Ryoichi Yamamoto
Feb. 20, 2023 13:14 English
-
- week2-3_The central limiting theorem
-
Ryoichi Yamamoto
Feb. 20, 2023 15:28 English
-
- week2-4_Random walk
-
Ryoichi Yamamoto
Feb. 20, 2023 13:07 English
-
- week3-1_Basic knowledge of Stochastic process
-
Ryoichi Yamamoto
Feb. 20, 2023 13:23 English
-
- week3-2_Brownian motion and the Langevin equation
-
Ryoichi Yamamoto
Feb. 20, 2023 14:20 English
-
- week3-3_The linear response theory and the Green-Kubo formula
-
Ryoichi Yamamoto
Feb. 20, 2023 11:28 English
-
- week4-1_Random force in the Langevin equation
-
Ryoichi Yamamoto
Feb. 20, 2023 09:01 English
-
- week4-2_Simple Python code to simulate Brownian motion
-
Ryoichi Yamamoto
Feb. 20, 2023 10:04 English
-
- week4-3_Simulations with on-the-fly animation
-
Ryoichi Yamamoto
Feb. 20, 2023 10:53 English
-
- week5-1_Distribution and time correlation
-
Ryoichi Yamamoto
Feb. 20, 2023 09:45 English
-
- week5-2_Mean square displacement and diffusion constant
-
Ryoichi Yamamoto
Feb. 20, 2023 08:59 English
-
- week5-3_Interacting Brownian particles_DTH
-
Ryoichi Yamamoto
Feb. 20, 2023 15:55 English
-
- week6-1_Time variations and distributions of real world processes
-
ohn J. Molina
Feb. 20, 2023 18:08 English
-
- week6-2_A Stochastic Dealer Model1
-
ohn J. Molina
Feb. 20, 2023 13:41 English
-
- week6-3_A Stochastic Dealer Model2
-
ohn J. Molina
Feb. 20, 2023 16:54 English
-
- week6-4_A Stochastic Dealer Model3
-
ohn J. Molina
Feb. 20, 2023 12:31 English
Details
- Faculty/
Graduate School - Graduate School of Engineering
- Note
- ■Sample Program https://youtube.com/playlist?list=PLD74lMZl_0Ga5e1UlD_ZqCjpykXR3a4dh
Syllabus
Instructor name | Instructors: Ryoichi Yamamoto & John J. Molina |
||||||||||||||||
---|---|---|---|---|---|---|---|---|---|---|---|---|---|---|---|---|---|
Outline and Purpose of the Course | The motion of falling leaves or small particles diffusing in a fluid is highly stochastic in nature. Therefore, such motions must be modeled as stochastic processes, for which exact predictions are no longer possible. This is in stark contrast to the deterministic motion of planets and stars, which can be perfectly predicted using celestial mechanics. This course is an introduction to stochastic processes through numerical simulations, with a focus on the proper data analysis needed to interpret the results. We will use the Jupyter (iPython) notebook as our programming environment. It is freely available for Windows, Mac, and Linux through the Anaconda Python Distribution. The students will first learn the basic theories of stochastic processes. Then, they will use these theories to develop their own python codes to perform numerical simulations of small particles diffusing in a fluid. Finally, they will analyze the simulation data according to the theories presented at the beginning of course. At the end of the course, we will analyze the dynamical data of more complicated systems, such as financial markets or meteorological data, using the basic theory of stochastic processes. |
||||||||||||||||
Course Goals |
|
||||||||||||||||
Schedule and Contents |
|
||||||||||||||||
Course Requirements | In addition to the knowledge of introductory physics, basic knowledge of linear algebra, calculus (differential and integral), and partial differential equations must be mastered beforehand. |
Related Courses
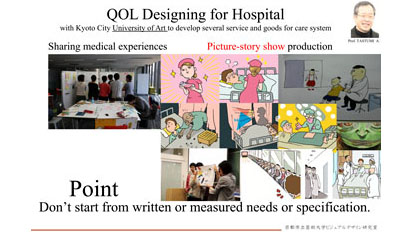
Naohide TOMITA
Graduate School of Engineering
2019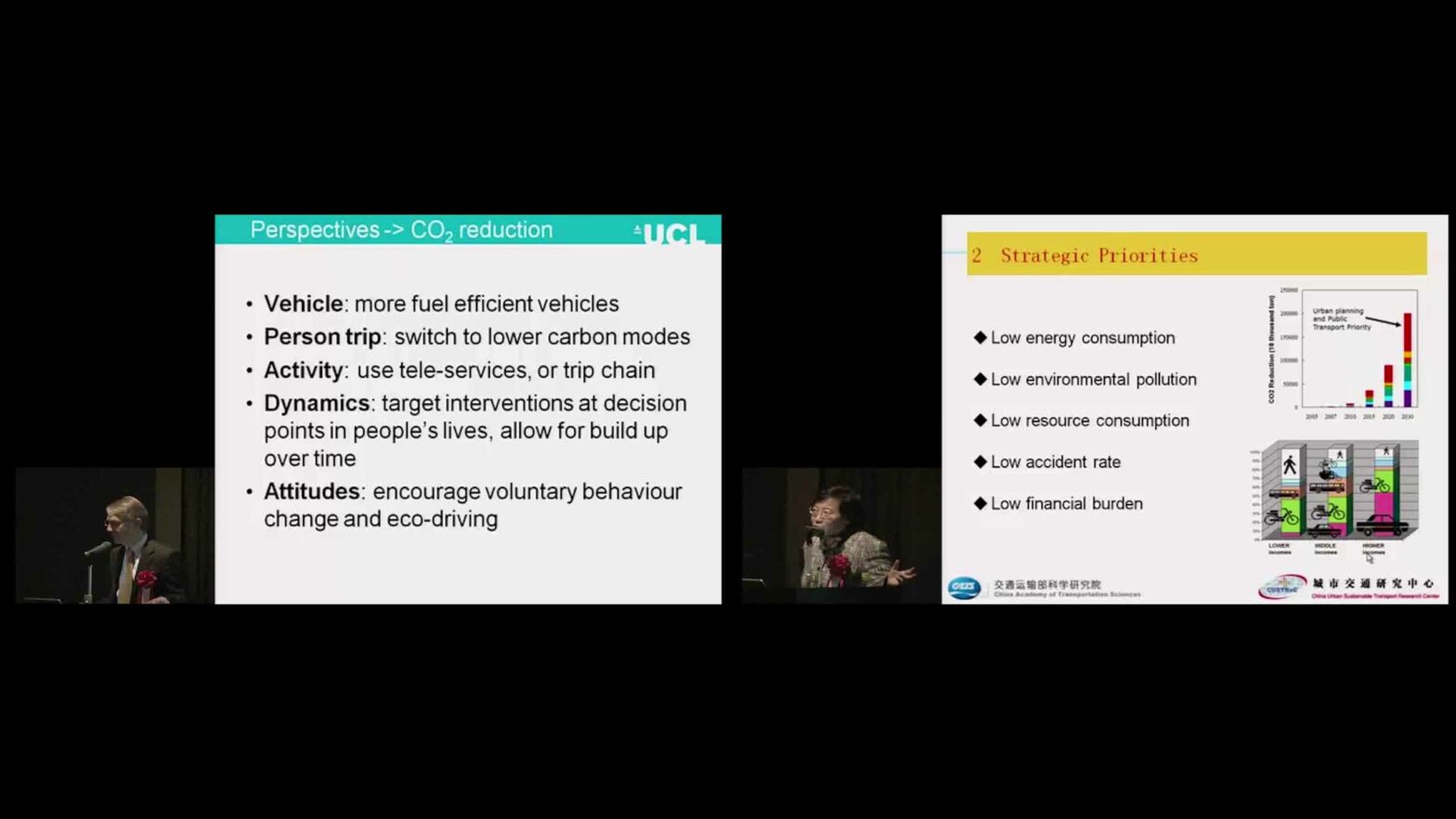
Graduate School of Engineering
2011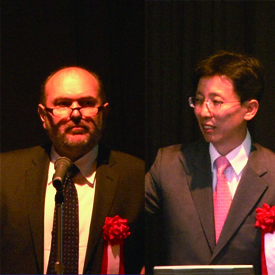
Mr. André von der Marck, Mr. Jaehak Oh
Graduate School of Engineering
2010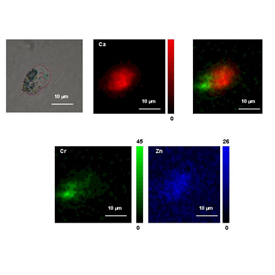
Ari IDE-EKTESSABI
Graduate School of Engineering